Smart pedagogy and smart learning objects
Smart pedagogy and smart learning objects
Smart pedagogy
The role of pedagogy in developing a smart education is important because there is an urgent need to find ways to teach and to support learning in the transformed learning environment. To support these changes, it must be acknowledged that pedagogy should change to become smart pedagogy, which has three clear dimensions:
1. Human developmental regularities, which include the conditions for the development of cognitive processes, the conditions for sensory development and the conditions for socio-emotional development
2. The taxonomy of the educational process, which includes the goals to be achieved and the regularities of the learning process necessary to achieve these goals
3. Technological progress, which entails the need for changes in teachers' pedagogical competence, one of the most important components of this competence being predictive analytical competence
Smart pedagogy (smart teaching and smart learning) can support educators in finding the answers on how to support learning in the transformed educational process, how to incorporate technologies into learning to support the development of metacognition, how to support knowledge building, how to support the development of digital competences and so on.
All of this indicates the necessity to ground the new direction of pedagogy, which is defined as smart pedagogy, to ensure smart use of different digital technologies.
Why Smart Pedagogy?
Reading Task 1: Study the following article:
Smart Pedagogy for Technology Enhanced Learning, edited by Linda Daniela, Springer, 2018 (Available in the learning resources folder)
Smart Learning Object and Content
Learning object is any entity, digital or non-digital, that can be used, re-used or referenced during technology-supported learning . Examples of learning object s include multimedia content, instructional content, instructional software and software tools that are referenced during technology-supported learning .
The term learning object has become more common in the eLearning industry. With the limited time that we have available for learning these days, there's an opportunity for students and working professionals to be able to take their education with them, learning incrementally.
How Does Learning Objects Work
Learning objects are meant to be simple to develop and implement. That's part of their appeal. The University of Sydney points out that the general idea behind them is threefold:
- Learning objects are self-contained, digital or non-digital resources that can be used for learning, education or training in bursts of time ranging from two to fifteen minutes.
- Learning objects can be stacked or grouped together to easily form collections of themed content, including traditionally designed courses.
- Learning objects are tagged with metadata to aid with search functionality.
Electronic learning object and content are forms of educational resources that forms the basis for smart learning object and content.
Learning objects are educational resources that can be employed in technology-supported learning. A learning object can be based on on electronic text, video clip, photograph, audio, graphic image, Java applet, simulation, a website, or any other resources that can be used in learning.
The traditional electronic learning content includes static text, images and videos on web pages, typically organized as hypertext, which links various pages together. Wikipedia and YouTube are typical examples of such content. Such electronic learning content has been usually organized for educational purposes using learning management systems, which can directly host content and provide links to external content. Electronic learning contents that incorporate interactive activities have included multiple choice questionnaires used for self-tests or quizzes. While these electronic learning contents have found use in all disciplines, in some disciplines such as computer science, educators have developed more elaborate learning resources that present dynamic and typically interactive learning content tailored to the discipline.
Robert J. Beck (Beck, 2008), suggests that learning objects have the following key characteristics:
- Learning objects are a new way of thinking about learning content. Traditionally, content comes in a several hour chunk. Learning objects are much smaller units of learning, typically ranging from 2 minutes to 15 minutes.
- Are self-contained - each learning object can be taken independently
- Are reusable - a single learning object may be used in multiple contexts for multiple purposes
- Can be aggregated - learning objects can be grouped into larger collections of content, including traditional course structures
- Are tagged with metadata - every learning object has descriptive information allowing it to be easily found by a search (Beck, 2008).
How to prepare a quiz in Powerpoint
https://youtu.be/Z50My6to14s
Components
The following is a list of some of the types of information that may be included in a learning object and its metadata:
- General Course Descriptive Data, including: course identifiers, language of content (English, Spanish, etc.), subject area (Maths, Reading, etc.), descriptive text, descriptive keywords
- Life Cycle, including: version, status
- Instructional Content, including: text, web pages, images, sound, video
- Glossary of Terms, including: terms, definition, acronyms
- Quizzes and Assessments, including: questions, answers
- Rights, including: cost, copyrights, restrictions on Use
- Relationships to Other Courses, including prerequisite courses
- Educational Level, including: grade level, age range, typical learning time, and difficulty. (IEEE 1484.12.1: 2002).
- Typology as defined by Churchill (2007): presentation, practice, simulation, conceptual models, information, and contextual representation (Churchill, 2007).
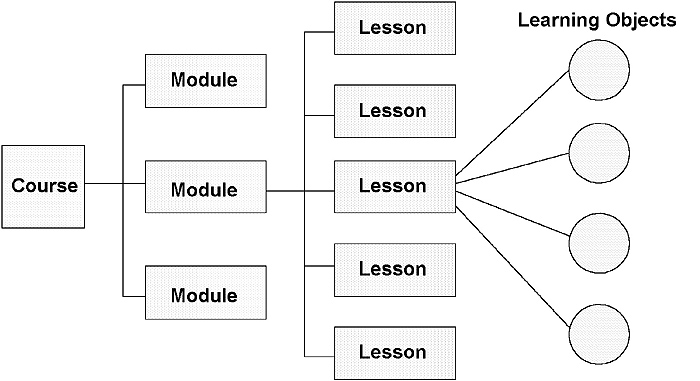
Figure: Learning objects hierarchy
Videos: What is a Learning Object?
Online learning object permits the followings:
Adaptability: instruction can be tailored to individual and situational needs.
Affordability: learning effectiveness can be significantly increased, while reducing time and costs.
Accessibility: instructional components can be accessed from one remote location and delivered to many other locations.
Reusability: instructional components can be incorporated into multiple applications.
Interoperability: instructional components can be developed in one location with one set of tools (or platform) or in another location with a different set of tools (or platform).
Durability: instructional components can be used when base technology changes, without the need for redesigning or recoding.
Retrievability: component can be retrieved when and where you want them.
Reliability: the other functions can be counted on to work when needed.
Assessability: pedagogical effectiveness, price, and usability can be assessed.
Discoverability: component can be easily found using simple understandable search terms.
Interchangeability: one component can be substituted for another.
Manageability: components can be handily found, inserted, replaced and substituted.
Mutability: a learning object that has been re-purposed and / or re-engineered, changed or simply re-used in some way different from its original intended design.
Contextualibility: a learning object that has been designed to have specific meaning and purpose to an intended learner.
Portability: how learning content can be easily loaded into a Learning Management System or other platforms.
(List expanded from Rory McGreal, 2005)
Learning object can be defined as any digital resource that is encapsulated in a lesson or assemblage of lessons grouped in units, modules, courses and even programs. A lesson can be defined as a piece of instruction, normally including a learning purposed or purposes.
Examples
NASA Science | Space Place - Explore Earth and Space
NASA Space Place's mission is to inspire and enrich upper-elementary-aged kids' learning of space and Earth science online through fun games, hands-on activities, informative articles and engaging short videos. With material and resources for parents and teachers, the NASA Space Place learning environment has something for everyone.
1. Reading Material -> Learning Object1
https://spaceplace.nasa.gov/moon-distance/en/
2. Educative Game Material - Learning Object 2
https://spaceplace.nasa.gov/satellite-insight/en/
3. Learning Activity - Learning Object 3
https://spaceplace.nasa.gov/topomap-clay/en/
4. Educative Video Material (Media) - Learning Object 4
https://spaceplace.nasa.gov/gps-pizza/en/
5. Play with Crafts - Learning Object 5
Learning object granularity
Online learning objects exists and interoperate at different levels of granularity. The simplest level is the content, information or knowledge object. This could be a simple text document, a photograph, a video clip, a 3D image, or any object that might be used for online learning. For example, a video clip from a melting ice from the North Pole would be an example of a simple media object. It becomes more useful to learners when a lesson is added to it. Many different lesson s can be created from one component. And many other subjects could be created from this one video clip.
Longer learning experiences or grouping of lessons are considered to be modules. A module normally comprises less than 10 hours of learning. When lessons are longer than 10 hours or if they consist of more than one module, they are considered to be a course. A group of courses that lead towards a certificate or diploma is considered to be a program.
These are all learning objects at different levels of granularity as shown in Figure below:
Figure: Learning objects at different levels of granularity
Metadata
In order to search for and find learning objects, which might be ideal for particular course, descriptions of their many characteristics are needed. This is what metadata do. they are essentially for addressing learning objects. Metadata are often described as being 'data about data'. A library card is a commonly known type of metadata. The author, title, ISBN code are all fields in this standard metadata format, shown in Figure 2.
The most important pieces of metadata typically associated with a learning object include:
- objective: The educational objective the learning object is instructing
- Prerequisites: The list of skills (typically represented as objectives) which the learner must know before viewing the learning object
- topic: Typically represented in a taxonomy, the topic the learning object is instructing
- interactivity: The Interaction Model of the learning object.
- technology requirements: The required system requirements to view the learning object.
The IEEE 1484.12.1 - 2002 Standard for Learning Object Metadata is an internationally recognized open standard (published by the Institute of Electrical and Electronics Engineers Standards Association, New York) for the description of learning objects. Relevant attributes of learning objects to be described include: type of object; author; owner; terms of distribution; format; and pedagogical attributes, such as teaching or interaction style.
IEEE Meta-data Elements and Structure
The elements and structure of the LOM conceptual data schema (some elements are of data types which give rise to leaf elements below the level of those shown in this diagram).https://www.imsglobal.org/metadata/mdv1p3/imsmd_bestv1p3.html
Figure: A schematic representation of the hierarchy of elements in the learning object metadata data model
Task 2: Follow the Youtube video as a simple example of taking some lesson content and converting it to a more interactive learning object using the PowerPoint software. Video:
Reading Task 3: Study the following research paper: Designing effective learning objects by Mohamed Ally (Available in the learning resources folder).
Case study: Increasing Adoption of Smart Learning Content (SLC) for Computer Science Education. Peter Brusilovsky, et al.
In order to build a big picture of such a multitude of tools and systems and how they are related to each other, as well as to traditional ELCs, we need to identify different characteristics of these SLCs. Here, we attempt a canonical description of their essential characteristics. We start by positing that some form of interactivity is a central aspect in all SLCs. Content that is presented to learners without any interaction is not smart learning content. For the purposes of our definition, interaction denotes exchange of data between the learner and the learning content and happens in the following three stages (see Figure 1 ).
1. Input is the data provided by the learner to the SLC. It is on a continuum from pre-specied (eg, playpause- rewind buttons for animation and visualization) to free-form (eg, code written by the learner, freeform input provided by the learner for visualization).
2. Process denotes how the SLC processes the input provided by the learner to select / generate output. If the SLC processes the input and generates / selects the output all on its own, it is fully computational. If on the other hand, the SLC merely facilitates the exchange of data between two or more human agents, it is not computational. The level of computational support is also a continuum from fully to none.
3. Output is the data provided by the SLC to the learner in response to the input. It is also on a continuum from generic, ie, the same output is provided regardless of the learner / context (eg, the same canned response to all learners: \ thank you for your submission ") to customized, ie, the output is customized to the learner and / or context (eg, whether the learner's answer is correct or not, the pages the learner must visit next based on prior performance of the learner).
Note that output entails the traditional notion of feedback, and customized output is what is traditionally referred to as adaptive feedback.
The level of smartness of learning content can now be characterized as being on a continuum from pre-specified input / generic output to free-form input / customized output:
- A non-computational tool with pre-specied input and generic output is not smart, eg, a traditional multiple-choice questionnaire (MCQ).
- A fully computational tool with free-form input, customized output is certainly smart, eg, an automatic essay evaluation system which gives tailored feedback on various aspects of a submitted essay.
The continuum described above can be presented as a three dimensional space with axes along Input dimension, Output dimension and Process dimension. Figure 2 illustrate the categorization of various online learning tools along these three dimensions or axes. To avoid confusion in presenting 3-dimensional data, the Process dimension in terms of two adjacent pictures where the left one describes non-computational content and the right one fully computational content. Most examples fall somewhere between these extremes. Note that the list of tools categorized in the figure is not meant to be comprehensive.
In Figure 2 :
- Hypertext is categorized as pre-specied input (learner's choices of hyperlinks are pre-specied) and generic output (pages do not change to suit the learner's needs). Adaptive hypertext also has pre-specied input, but the output is more customized than in the case of hypertext, because the selection and presentation of pages is based on learner interaction.
- Online MCQ without feedback is non-computational, has pre-specied input, and generic output (such as \ thank you, your responses were received "). Online MCQ that provides summative feedback is computational and more customized. Online MCQ that provides formative feedback and allows the learner to review his / her answer is more computational with more customized output.
Figure 2 : Examples of learning content classified by using input (horizontal axis), process and output (vertical axis) dimensions. Note, the left and right figures present the two extremes of the process dimension. For clarity, other values on the process dimension are not visualized. Note also that the items are simple some selected examples to highlight to categorization system, and their positions in the graph only approximately reflect their value within the two other axes
- Automated program assessment is fully computational, with free-form input (code), and somewhat customized output (based on the code written by the learner). Summative (only whether the program is correct or not) and formative feedback (feedback including why the program is incorrect) are on different parts of the output continuum.
- Algorithm visualization is fully computational (unless made manually by the user). Its input could range from fixed input data manipulation to free-form user input data, the latter including manipulation of the underlying code itself. The same applies to program visualization.
- Algorithm and program simulation exercises are computational (supported by the tool, although the learner manipulates the provided visualization). The input is pre-specied and the output is moderately customized.
Finally, in order to provide customized output, smart learning contents must collect data about the learner's interactions. They may save the collected data to display the learner's progress, provide suspend-and-resume capability to the learner, generate reports for the teacher and provide analytic capabilities to the author of the smart learning content.
References and further readings:
Online education using learning objects. edited by Rory McGreal. Routledge 2005
Didactics of Smart Pedagogy - Smart Pedagogy for Technology Enhanced Learning. Editor: Daniela, Linda (Ed.). Springer ISBN 978-3-030-01551-0
B. Pandya, K. Shah 'SMART' Pedagogy to manage invasion of Smartphones in m-campus.
Beck, Robert J., "What Are Learning Objects?", Learning Objects, Center for International Education, University of Wisconsin-Milwaukee, retrieved 2008-04-29.
Churchill, D. (2007). Towards a useful classification of learning objects. Educational Technology Research & Development, 55 (5), 479-497.